NanoString
Deep Learning-Based Localization for RNA Spatial Transcriptomics
Nanostring spatial molecular imaging platform (SMI) leverages single molecule hybridization-based sequencing chemistry to spatially profile expression of 1000+ genes at single cell and sub cellular resolution across tissue samples. The current RNA localization algorithm relies on commonly used methodologies where potential target locations are segmented from raw images and fit with a function that approximates a point spread function. While efficient and fast, this method will fail in the multi-emitter regime. Due to the desire to increase plexity and/or use reduced codesets, it is critical to evaluate other localization schemes that are designed for densely packed data. DECODE is a state-of-the-art deep learning-based approach that enables high precision localization microscopy in dense images. This student team worked to deliver modified DECODE modules that are tailored towards high density SMI samples. The student team also worked to develop models with adequate consideration towards compensating raw data that has highly variable background and large signal dynamic ranges and outperforms standard localization methods in SMI data by an order of magnitude with respect to density, defined as having a Jaccard index >~70%. The student team also worked to provide a Python module to enable 3D clustering of localizations to find the best fit Z-plane to allow for code matching via Nanostring’s matching module.
Faculty Adviser
Payman Arabshahi,
Associate Professor, UW ECE,
Electrical & Computer Engineering
Students
Kyle Herbruger
Meghan Schmidt
Michael Bargenda
Pavan Kuman Anand
Shreemit Garimella
Zhenghang Wang
Related News
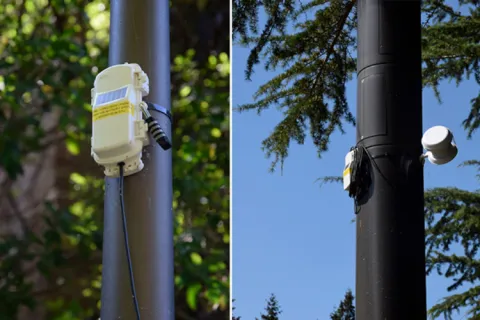
Fri, 09/20/2024 | UW Civil & Environmental Engineering
Smarter irrigation for a greener UW
A new project combines satellite data with ground sensors to conserve water and create a more sustainable campus environment.
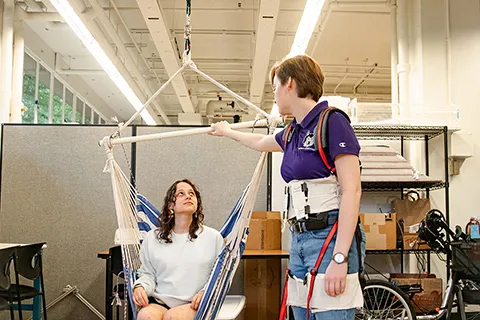
Mon, 09/09/2024 | UW Mechanical Engineering
Testing an in-home mobility system
Through innovative capstone projects, engineering students worked with community members on an adaptable mobility system.
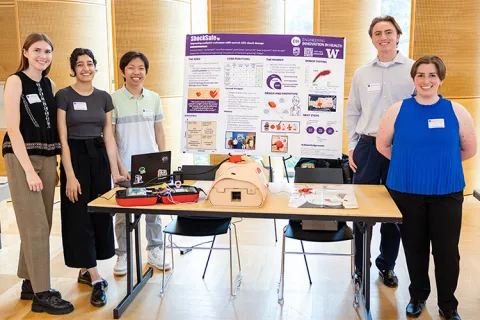
Mon, 08/19/2024 | UW Mechanical Engineering
Students strive to ensure accurate AED shock dosage
ShockSafe, developed by students with the help of mentors from Philips and Engineering Innovation in Health (EIH), can distinguish between children and adults during cardiac arrest emergencies.
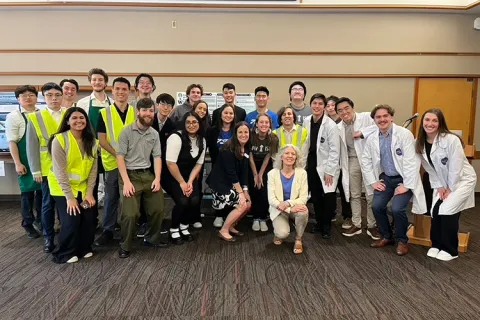
Wed, 08/07/2024 | Snohomish County News
Snohomish County, University of Washington partnership boosts efficiency in enterprise scanning center
UW Industrial and Systems Engineering Capstone Project set to save Snohomish County over $40,000 annually.